Matplotlib의 여러가지 플롯#
Matplotlib는 기본적인 라인 플롯 이외에도 다양한 챠트/플롯 유형을 지원한다.
바 차트#
x 데이터가 카테고리 값인 경우에는 bar
명령과 barh
명령으로 바 차트(bar chart) 시각화를 할 수 있다. 가로 방향으로 바 차트를 그리려면 barh
명령을 사용한다.
자세한 내용은 다음 웹사이트를 참조한다.
http://matplotlib.org/api/pyplot_api.html#matplotlib.pyplot.bar
http://matplotlib.org/api/pyplot_api.html#matplotlib.pyplot.barh
바 차트 작성시 주의점은 첫번째 인수인 left
가 x축에서 바(bar)의 왼쪽 변의 위치를 나타낸다는 점이다.
import matplotlib as mpl
import matplotlib.pylab as plt
y = [2, 3, 1]
x = np.arange(len(y))
xlabel = ['가', '나', '다']
plt.title("Bar Chart")
plt.bar(x, y)
plt.xticks(x, xlabel)
plt.yticks(sorted(y))
plt.xlabel("가나다")
plt.ylabel("빈도 수")
plt.show()
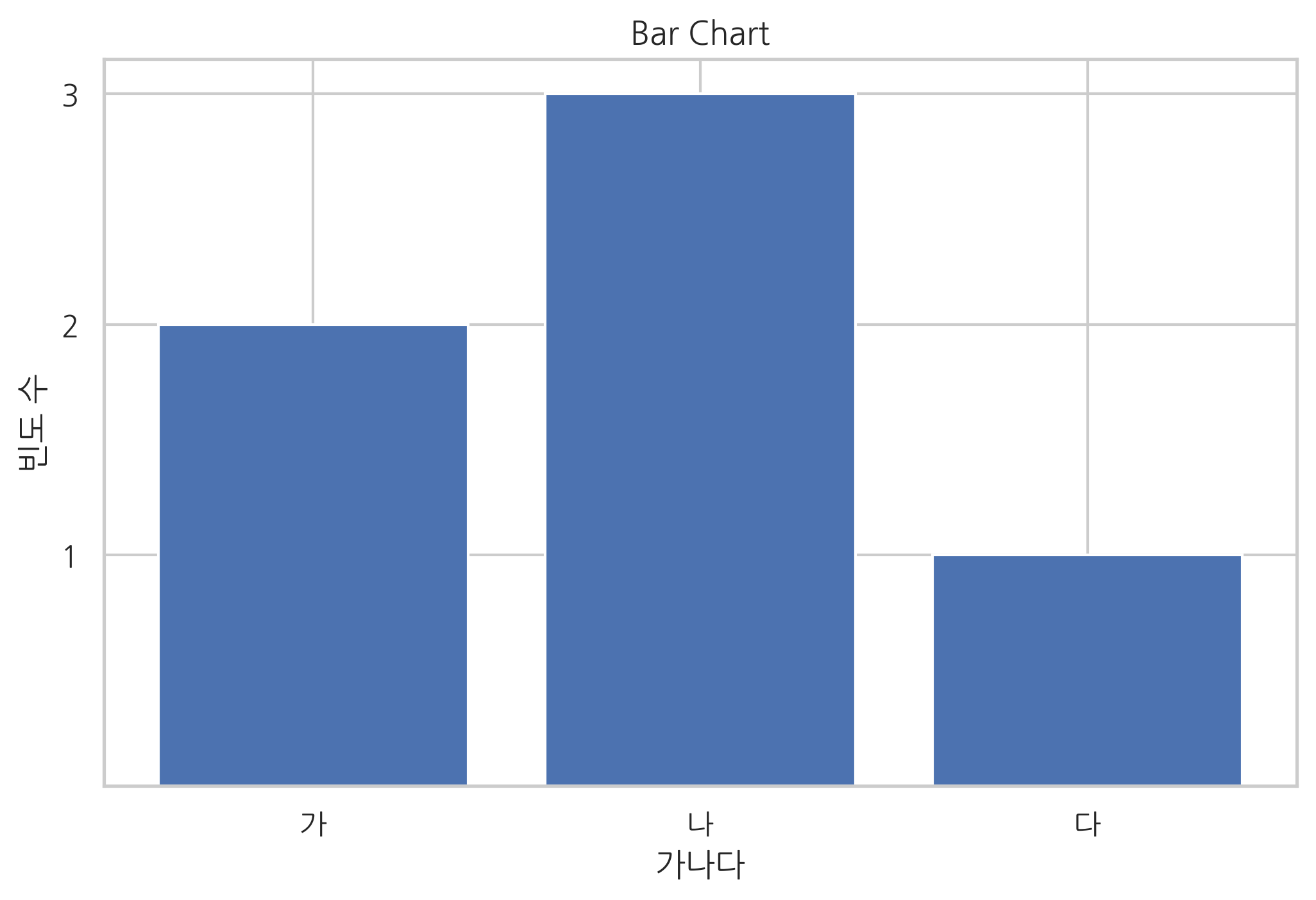
xerr
인수나 yerr
인수를 지정하면 에러 바(error bar)를 추가할 수 있다.
다음 코드에서 alpha
는 투명도를 지정한다. 0이면 완전 투명, 1이면 완전 불투명이다.
np.random.seed(0)
people = ['몽룡', '춘향', '방자', '향단']
y_pos = np.arange(len(people))
performance = 3 + 10 * np.random.rand(len(people))
error = np.random.rand(len(people))
plt.title("Barh Chart")
plt.barh(y_pos, performance, xerr=error, alpha=0.4)
plt.yticks(y_pos, people)
plt.xlabel('x 라벨')
plt.show()
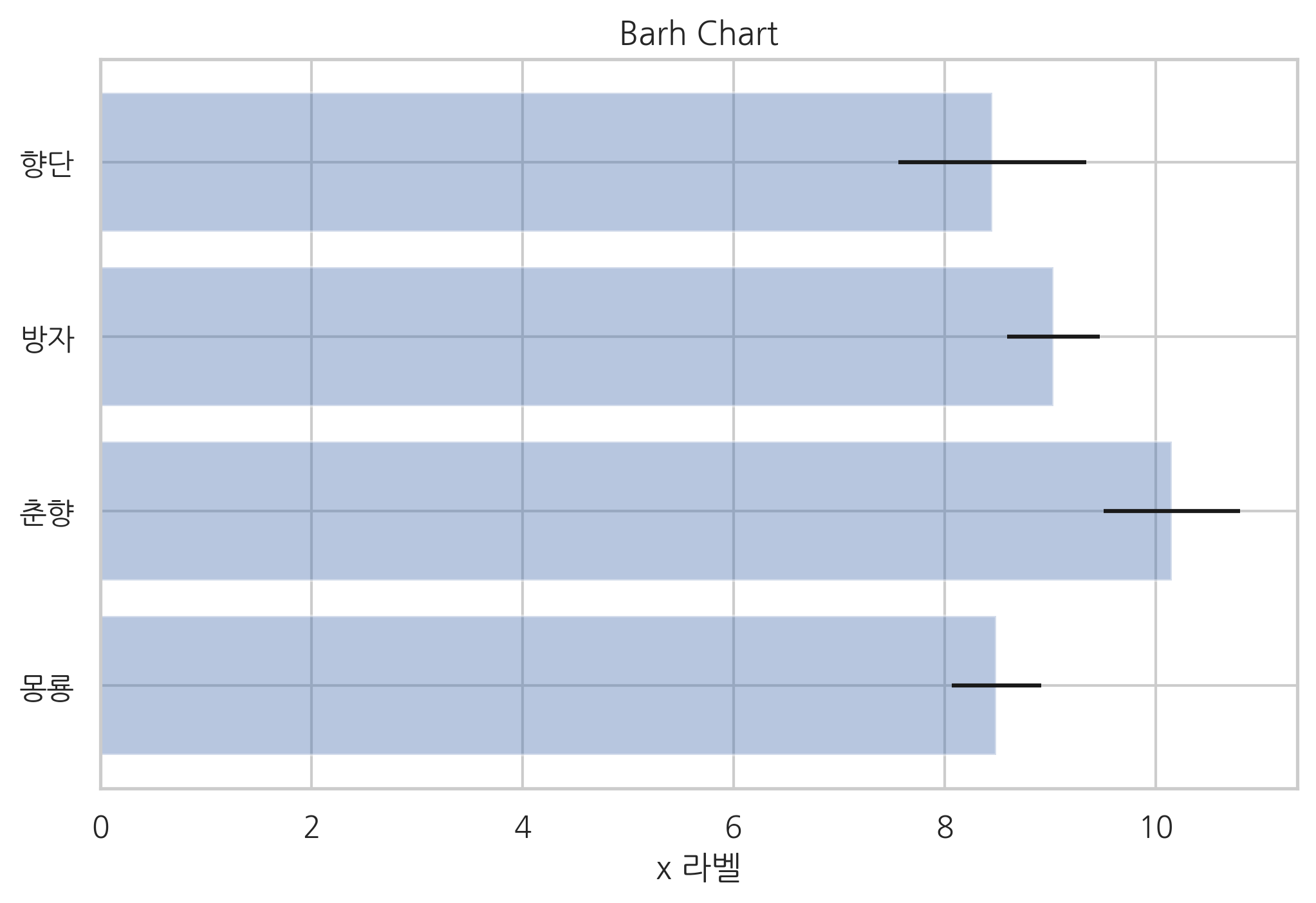
스템 플롯#
바 차트와 유사하지만 폭(width)이 없는 스템 플롯(stem plot)도 있다. 주로 이산 확률 함수나 자기상관관계(auto-correlation)를 묘사할 때 사용된다.
x = np.linspace(0.1, 2 * np.pi, 10)
plt.title("Stem Plot")
plt.stem(x, np.cos(x), '-.')
plt.show()
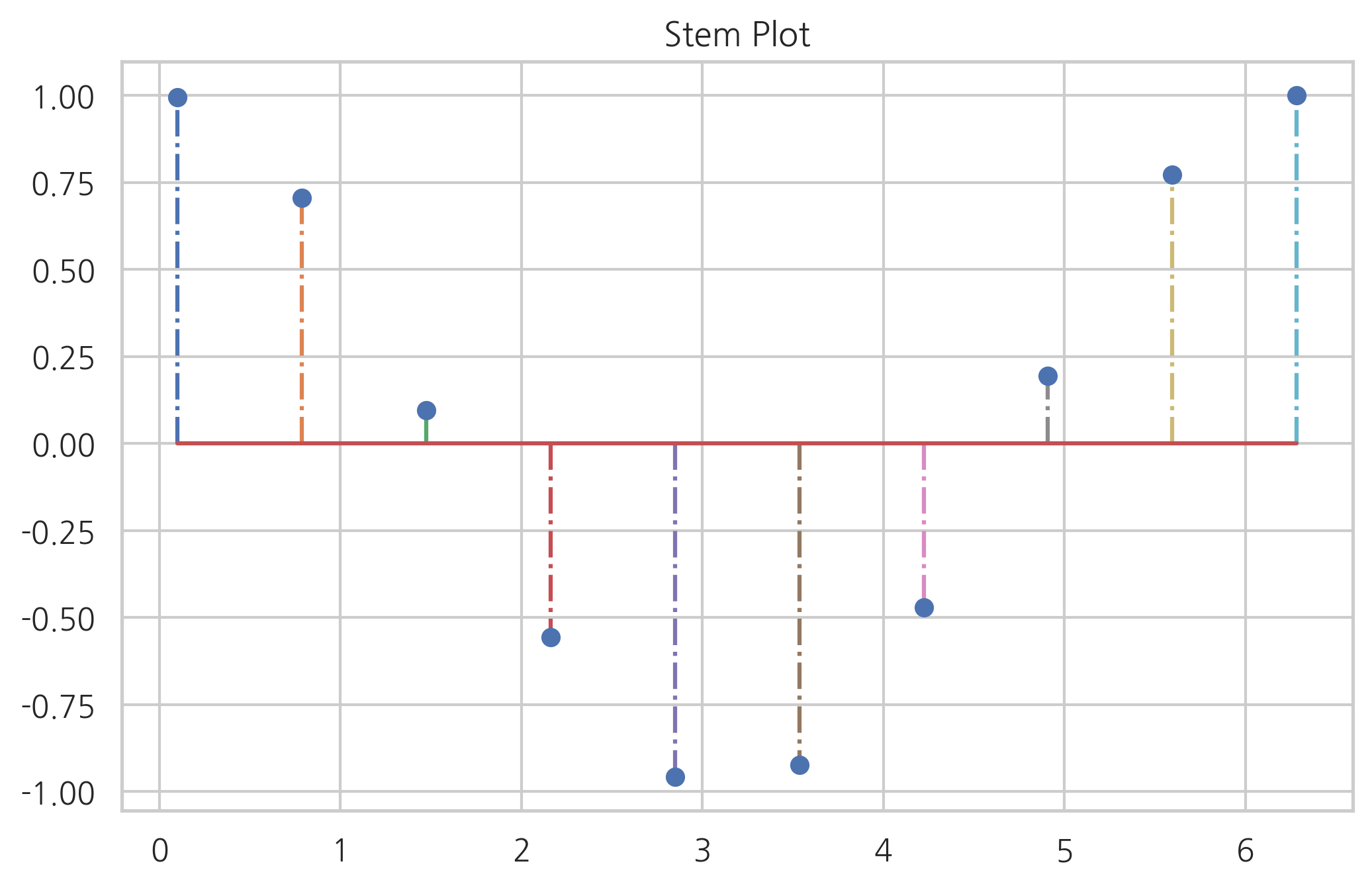
파이 차트#
카테고리 별 값의 상대적인 비교를 해야 할 때는 pie
명령으로 파이 차트(pie chart)를 그릴 수 있다. 파이 차트를 그릴 때는 윈의 형태를 유지할 수 있도록 다음 명령을 실행해야 한다.
plt.axis('equal')
자세한 내용은 다음을 참조한다.
labels = ['개구리', '돼지', '개', '통나무']
sizes = [15, 30, 45, 10]
colors = ['yellowgreen', 'gold', 'lightskyblue', 'lightcoral']
explode = (0, 0.1, 0, 0)
plt.title("Pie Chart")
plt.pie(sizes, explode=explode, labels=labels, colors=colors,
autopct='%1.1f%%', shadow=True, startangle=90)
plt.axis('equal')
plt.show()
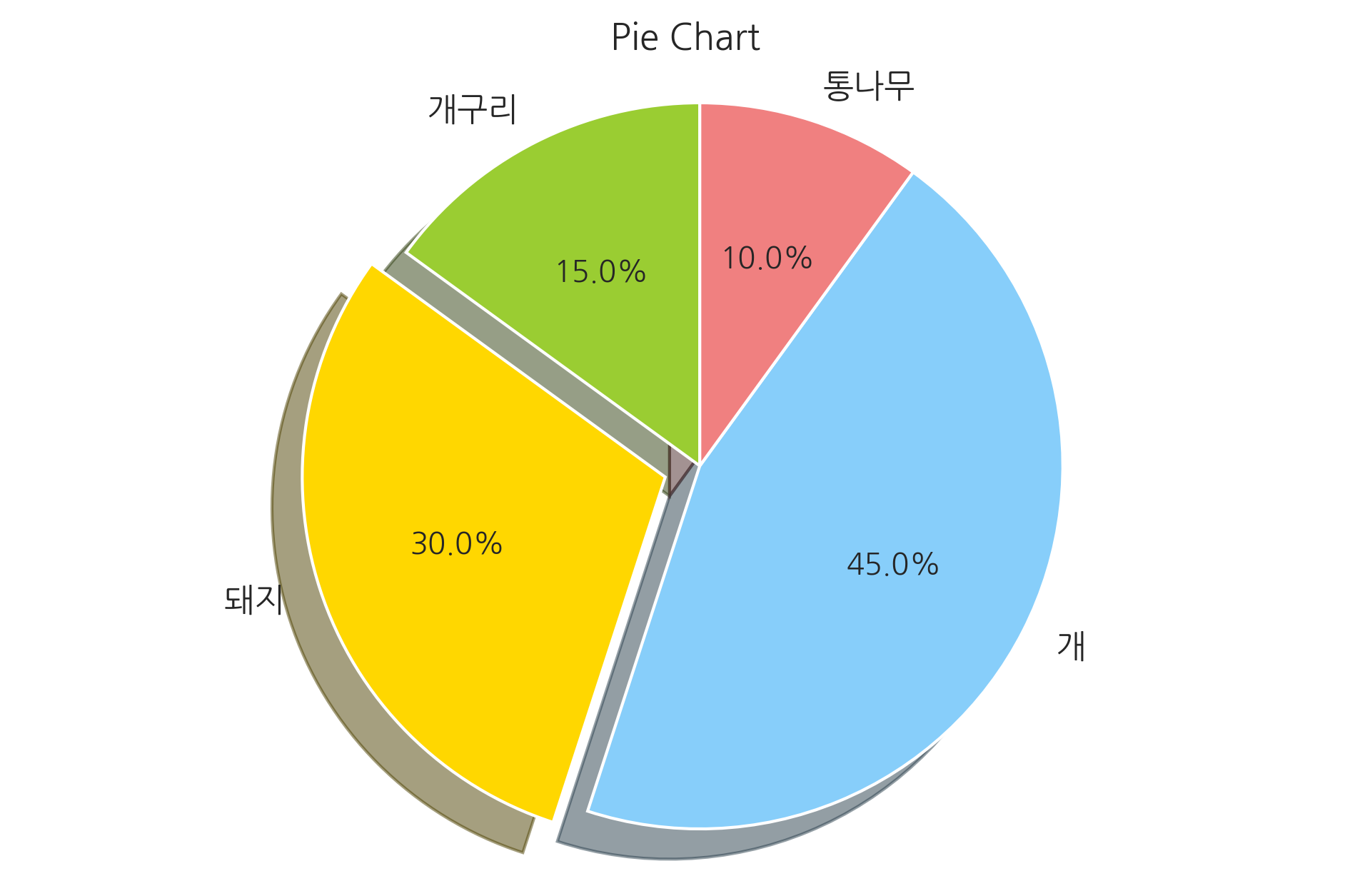
히스토그램#
히스토그램을 그리기 위한 hist
명령도 있다. hist
명령은 bins
인수로 데이터를 집계할 구간 정보를 받는다. 반환값으로 데이터 집계 결과를 반환한다.
np.random.seed(0)
x = np.random.randn(1000)
plt.title("Histogram")
arrays, bins, patches = plt.hist(x, bins=10)
plt.show()
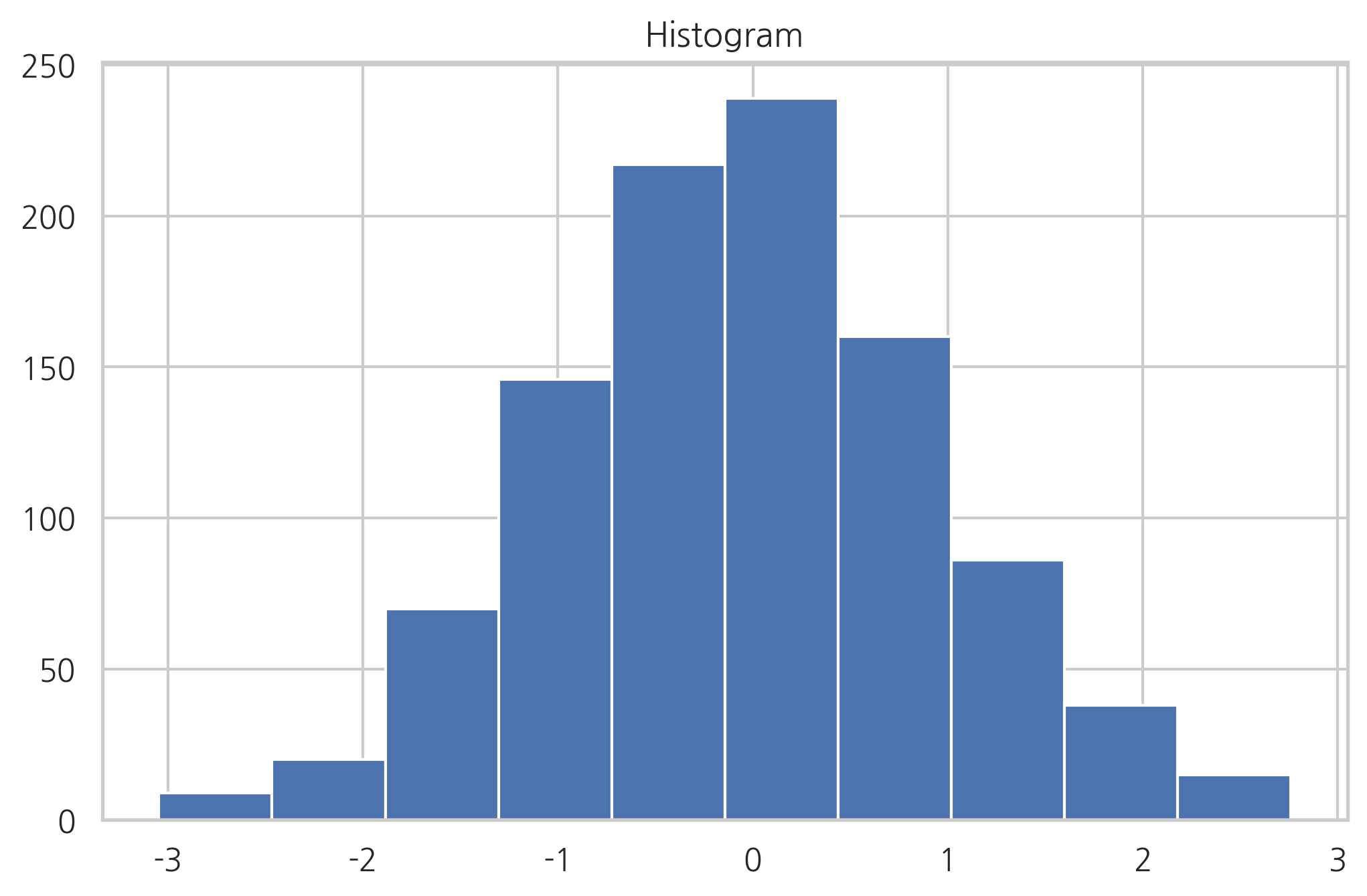
arrays
array([ 9., 20., 70., 146., 217., 239., 160., 86., 38., 15.])
bins
array([-3.04614305, -2.46559324, -1.88504342, -1.3044936 , -0.72394379,
-0.14339397, 0.43715585, 1.01770566, 1.59825548, 2.1788053 ,
2.75935511])
스캐터 플롯#
2차원 데이터 즉, 두 개의 실수 데이터 집합의 상관관계를 살펴보려면 scatter
명령으로 스캐터 플롯을 그린다. 스캐터 플롯의 점 하나의 위치는 데이터 하나의 x, y 값이다.
np.random.seed(0)
X = np.random.normal(0, 1, 100)
Y = np.random.normal(0, 1, 100)
plt.title("Scatter Plot")
plt.scatter(X, Y)
plt.show()
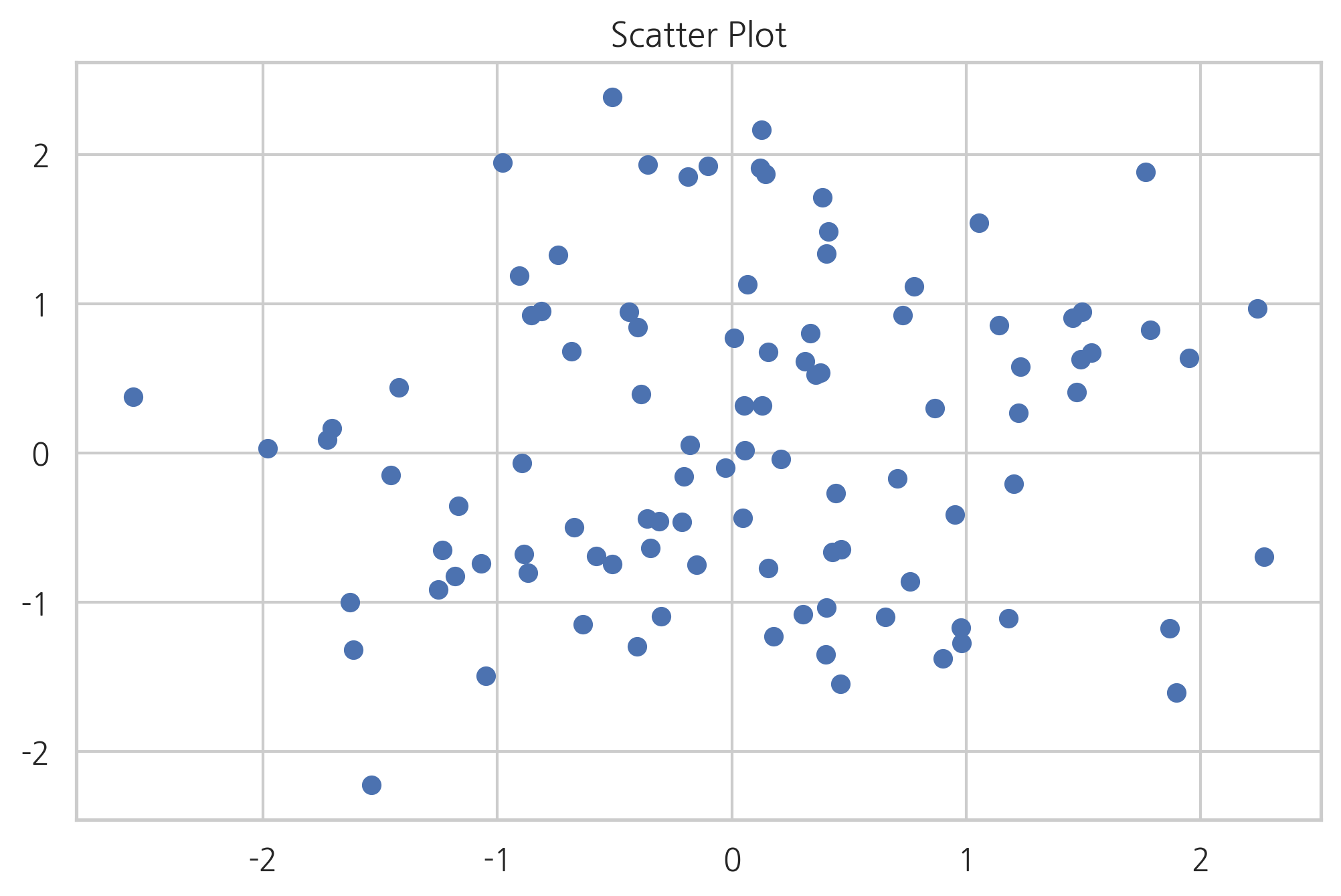
데이터가 2차원이 아니라 3차원 혹은 4차원인 경우에는 점 하나의 크기 혹은 색깔을 이용하여 다른 데이터 값을 나타낼 수도 있다. 이런 차트를 버블 차트(bubble chart)라고 한다. 크기는 s
인수로 색깔은 c
인수로 지정한다.
N = 30
np.random.seed(0)
x = np.random.rand(N)
y1 = np.random.rand(N)
y2 = np.random.rand(N)
y3 = np.pi * (15 * np.random.rand(N))**2
plt.title("Bubble Chart")
plt.scatter(x, y1, c=y2, s=y3)
plt.show()
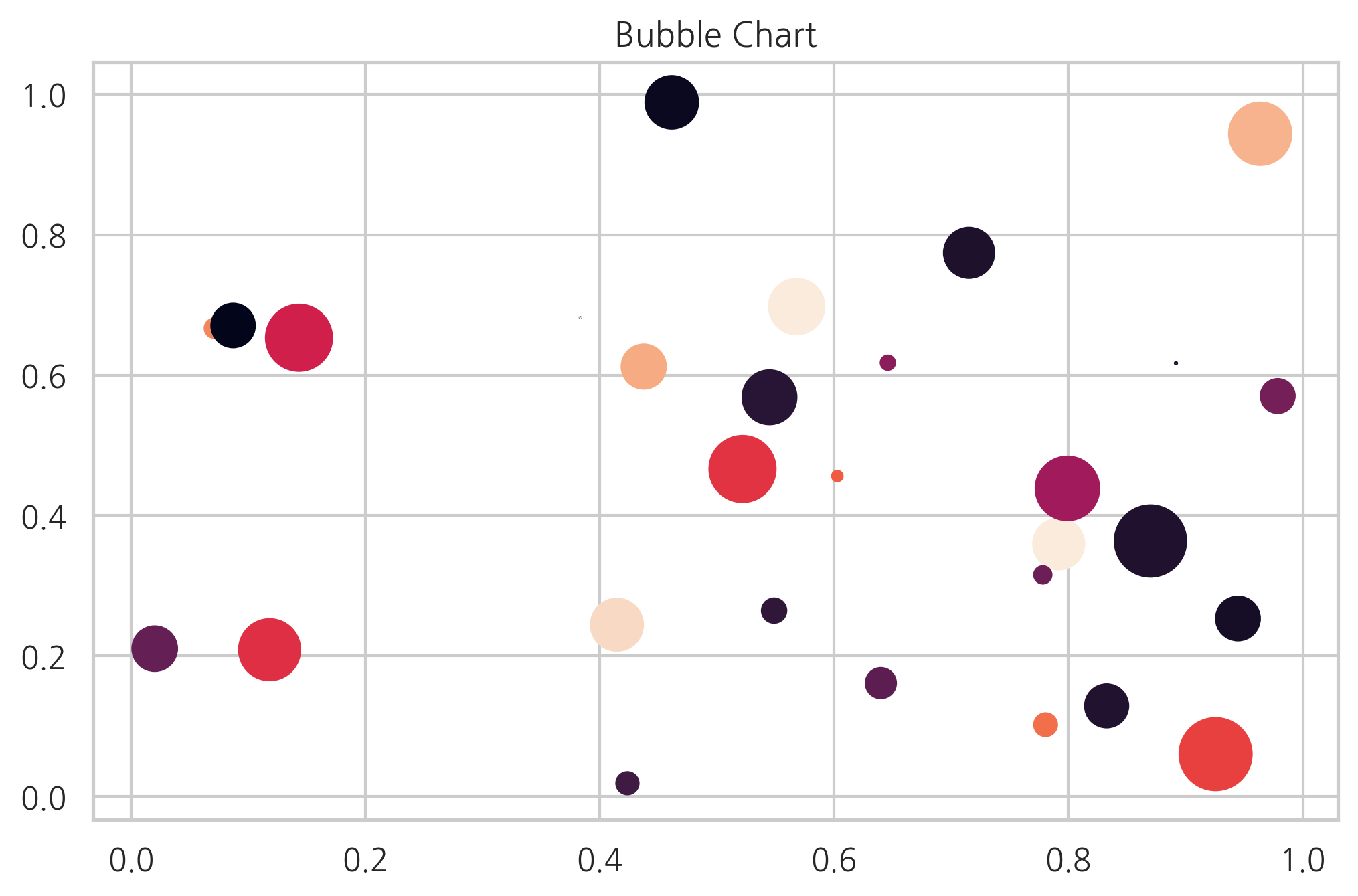
Imshow#
화상(image) 데이터처럼 행과 열을 가진 행렬 형태의 2차원 데이터는 imshow
명령을 써서 2차원 자료의 크기를 색깔로 표시하는 것이다.
from sklearn.datasets import load_digits
digits = load_digits()
X = digits.images[0]
X
array([[ 0., 0., 5., 13., 9., 1., 0., 0.],
[ 0., 0., 13., 15., 10., 15., 5., 0.],
[ 0., 3., 15., 2., 0., 11., 8., 0.],
[ 0., 4., 12., 0., 0., 8., 8., 0.],
[ 0., 5., 8., 0., 0., 9., 8., 0.],
[ 0., 4., 11., 0., 1., 12., 7., 0.],
[ 0., 2., 14., 5., 10., 12., 0., 0.],
[ 0., 0., 6., 13., 10., 0., 0., 0.]])
plt.title("mnist digits; 0")
plt.imshow(X, interpolation='nearest', cmap=plt.cm.bone_r)
plt.xticks([])
plt.yticks([])
plt.grid(False)
plt.subplots_adjust(left=0.35, right=0.65, bottom=0.35, top=0.65)
plt.show()
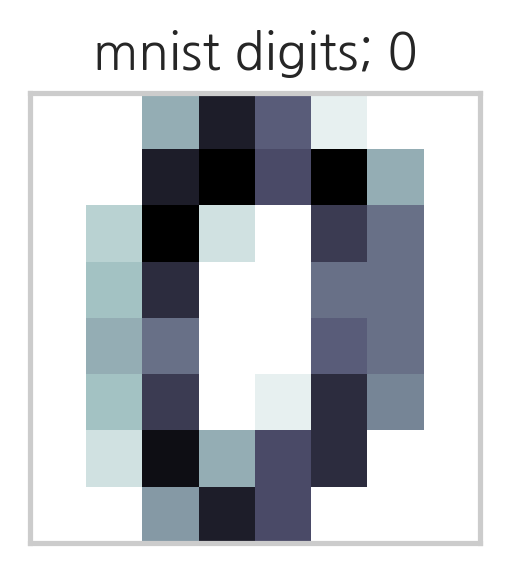
데이터 수치를 색으로 바꾸는 함수는 칼라맵(color map)이라고 한다. 칼라맵은 cmap
인수로 지정한다. 사용할 수 있는 칼라맵은 plt.cm
의 속성으로 포함되어 있다. 아래에 일부 칼라맵을 표시하였다. 칼라맵은 문자열로 지정해도 된다. 칼라맵에 대한 자세한 내용은 다음 웹사이트를 참조한다.
dir(plt.cm)[:10]
['Accent',
'Accent_r',
'Blues',
'Blues_r',
'BrBG',
'BrBG_r',
'BuGn',
'BuGn_r',
'BuPu',
'BuPu_r']
fig, axes = plt.subplots(1, 4, figsize=(12, 3),
subplot_kw={'xticks': [], 'yticks': []})
axes[0].set_title("plt.cm.Blues")
axes[0].imshow(X, interpolation='nearest', cmap=plt.cm.Blues)
axes[1].set_title("plt.cm.Blues_r")
axes[1].imshow(X, interpolation='nearest', cmap=plt.cm.Blues_r)
axes[2].set_title("plt.BrBG")
axes[2].imshow(X, interpolation='nearest', cmap='BrBG')
axes[3].set_title("plt.BrBG_r")
axes[3].imshow(X, interpolation='nearest', cmap='BrBG_r')
plt.show()
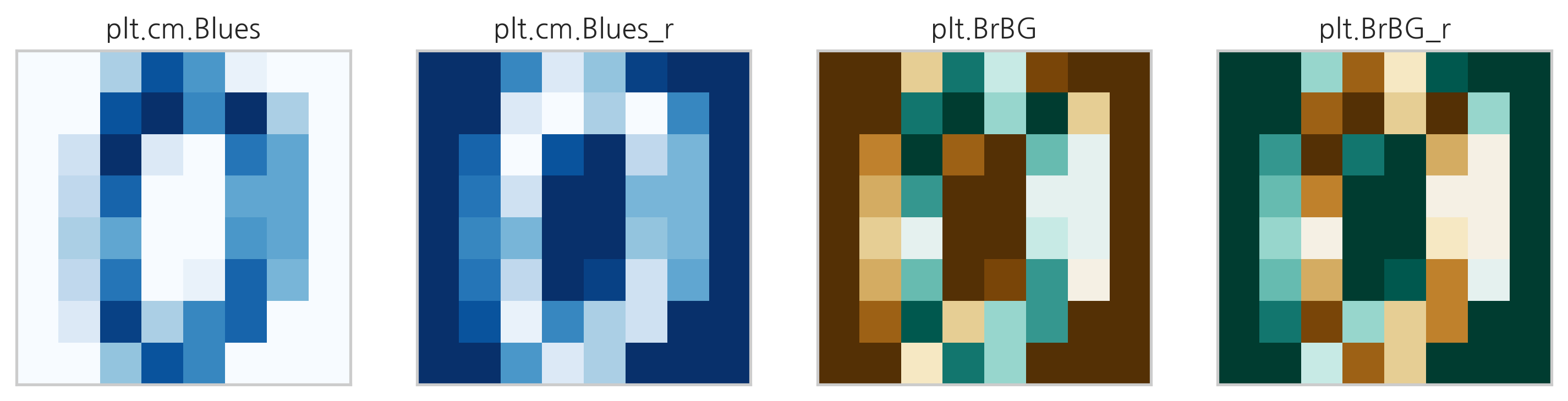
imshow
명령은 자료의 시각화를 돕기위해 다양한 2차원 인터폴레이션을 지원한다.
methods = [
None, 'none', 'nearest', 'bilinear', 'bicubic', 'spline16',
'spline36', 'hanning', 'hamming', 'hermite', 'kaiser', 'quadric',
'catrom', 'gaussian', 'bessel', 'mitchell', 'sinc', 'lanczos'
]
fig, axes = plt.subplots(3, 6, figsize=(12, 6),
subplot_kw={'xticks': [], 'yticks': []})
for ax, interp_method in zip(axes.flat, methods):
ax.imshow(X, cmap=plt.cm.bone_r, interpolation=interp_method)
ax.set_title(interp_method)
plt.show()
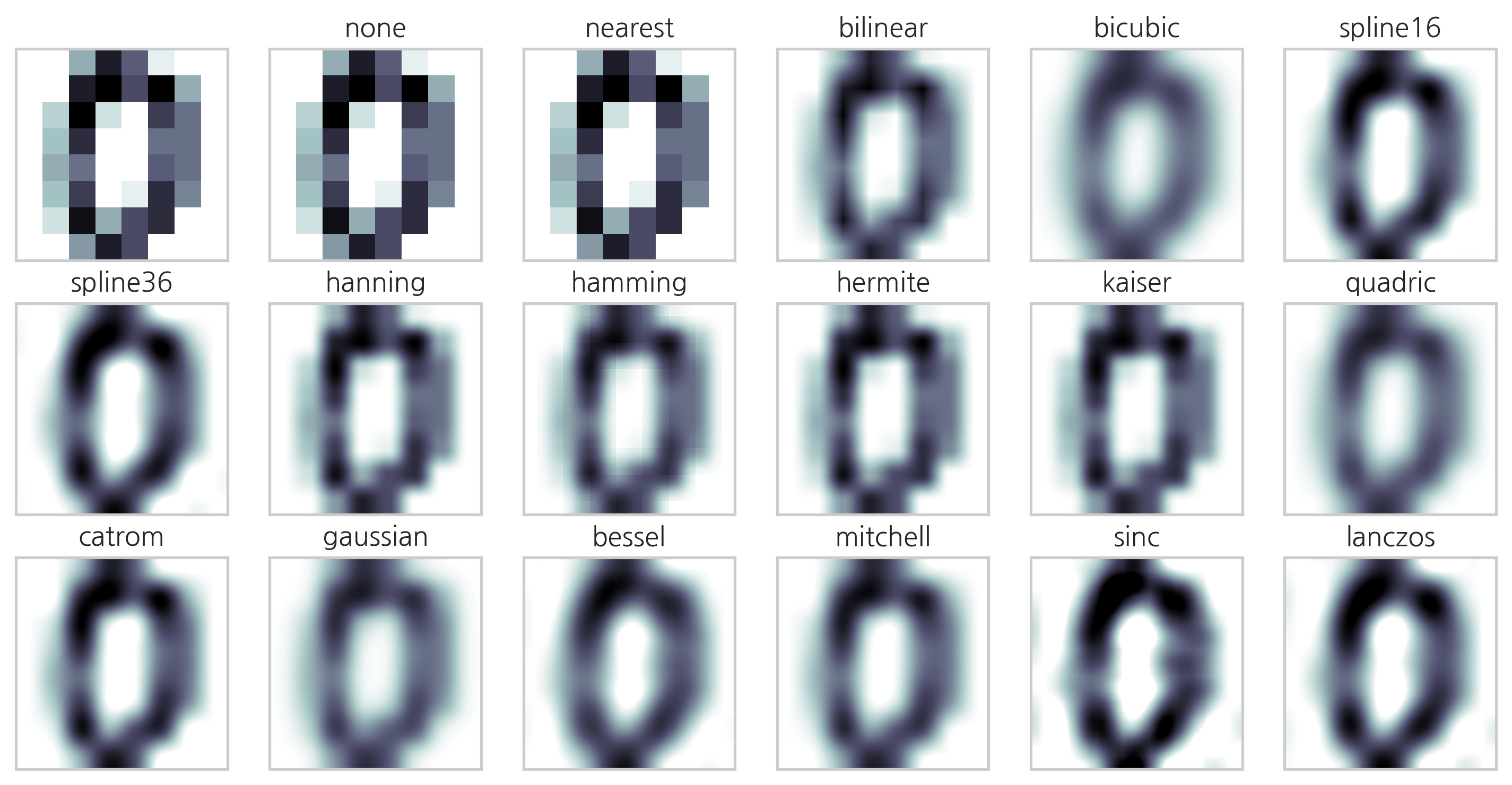
컨투어 플롯#
입력 변수가 x, y 두 개이고 출력 변수가 z 하나인 경우에는 3차원 자료가 된다. 3차원 자료를 시각화하는 방법은 명암이 아닌 등고선(contour)을 사용하는 방법이다. contour
혹은 contourf
명령을 사용한다. contour
는 등고선만 표시하고 contourf
는 색깔로 표시한다. 입력 변수 x, y는 그대로 사용할 수 없고 meshgrid
명령으로 그리드 포인트 행렬을 만들어야 한다. 더 자세한 내용은 다음 웹사이트를 참조한다.
http://matplotlib.org/api/pyplot_api.html#matplotlib.pyplot.contour
http://matplotlib.org/api/pyplot_api.html#matplotlib.pyplot.contourf
def f(x, y):
return (1 - x / 2 + x ** 5 + y ** 3) * np.exp(-x ** 2 - y ** 2)
n = 256
x = np.linspace(-3, 3, n)
y = np.linspace(-3, 3, n)
XX, YY = np.meshgrid(x, y)
ZZ = f(XX, YY)
plt.title("Contour plots")
plt.contourf(XX, YY, ZZ, alpha=.75, cmap='jet')
plt.contour(XX, YY, ZZ, colors='black')
plt.show()
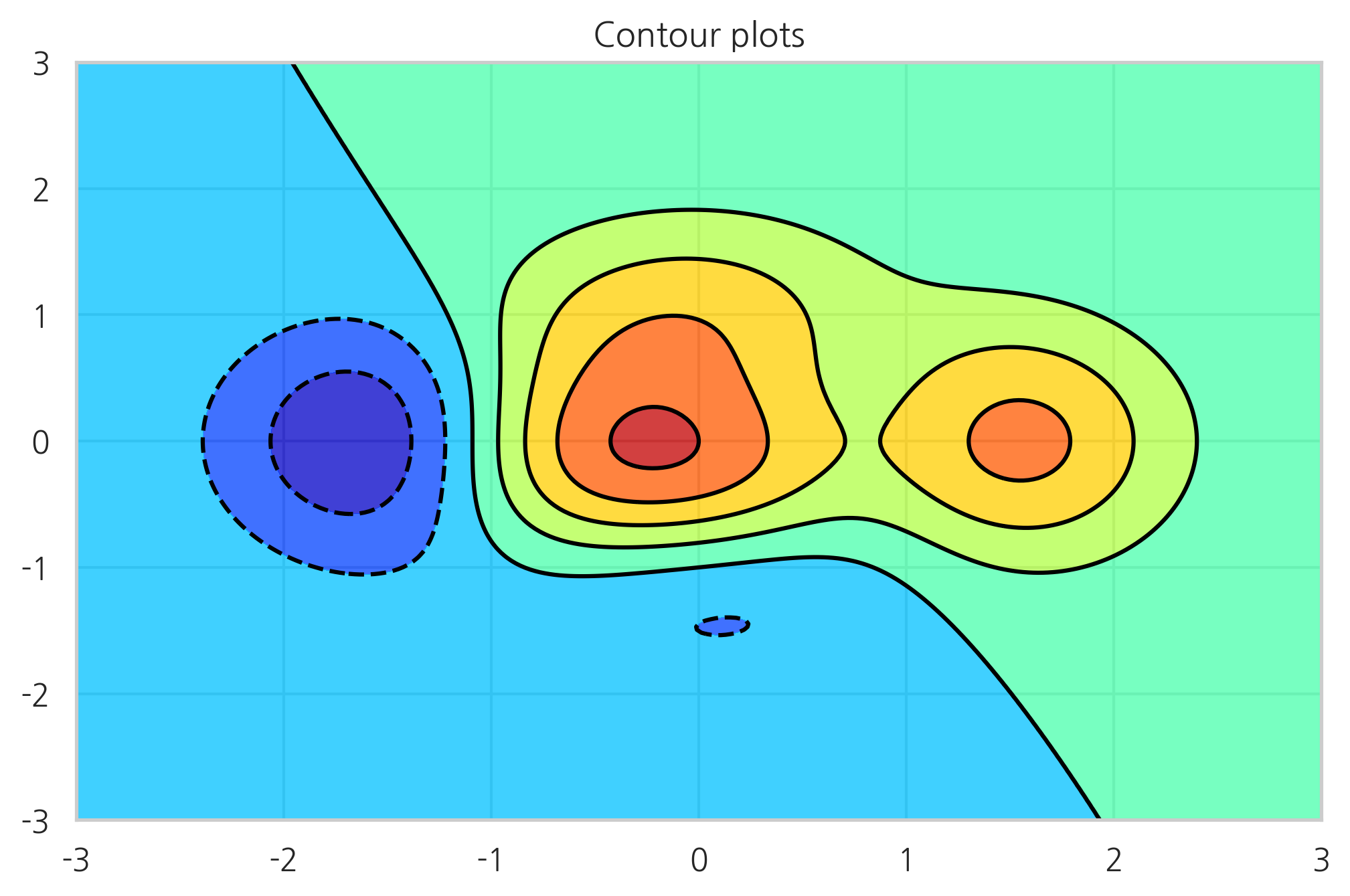
3D 서피스 플롯#
3차원 플롯은 등고선 플롯과 달리 Axes3D
라는 3차원 전용 axes를 생성하여 입체적으로 표시한다.
plot_wireframe
, plot_surface
명령을 사용한다.
from mpl_toolkits.mplot3d import Axes3D
X = np.arange(-4, 4, 0.25)
Y = np.arange(-4, 4, 0.25)
XX, YY = np.meshgrid(X, Y)
RR = np.sqrt(XX**2 + YY**2)
ZZ = np.sin(RR)
fig = plt.figure()
ax = Axes3D(fig)
ax.set_title("3D Surface Plot")
ax.plot_surface(XX, YY, ZZ, rstride=1, cstride=1, cmap='hot')
plt.show()
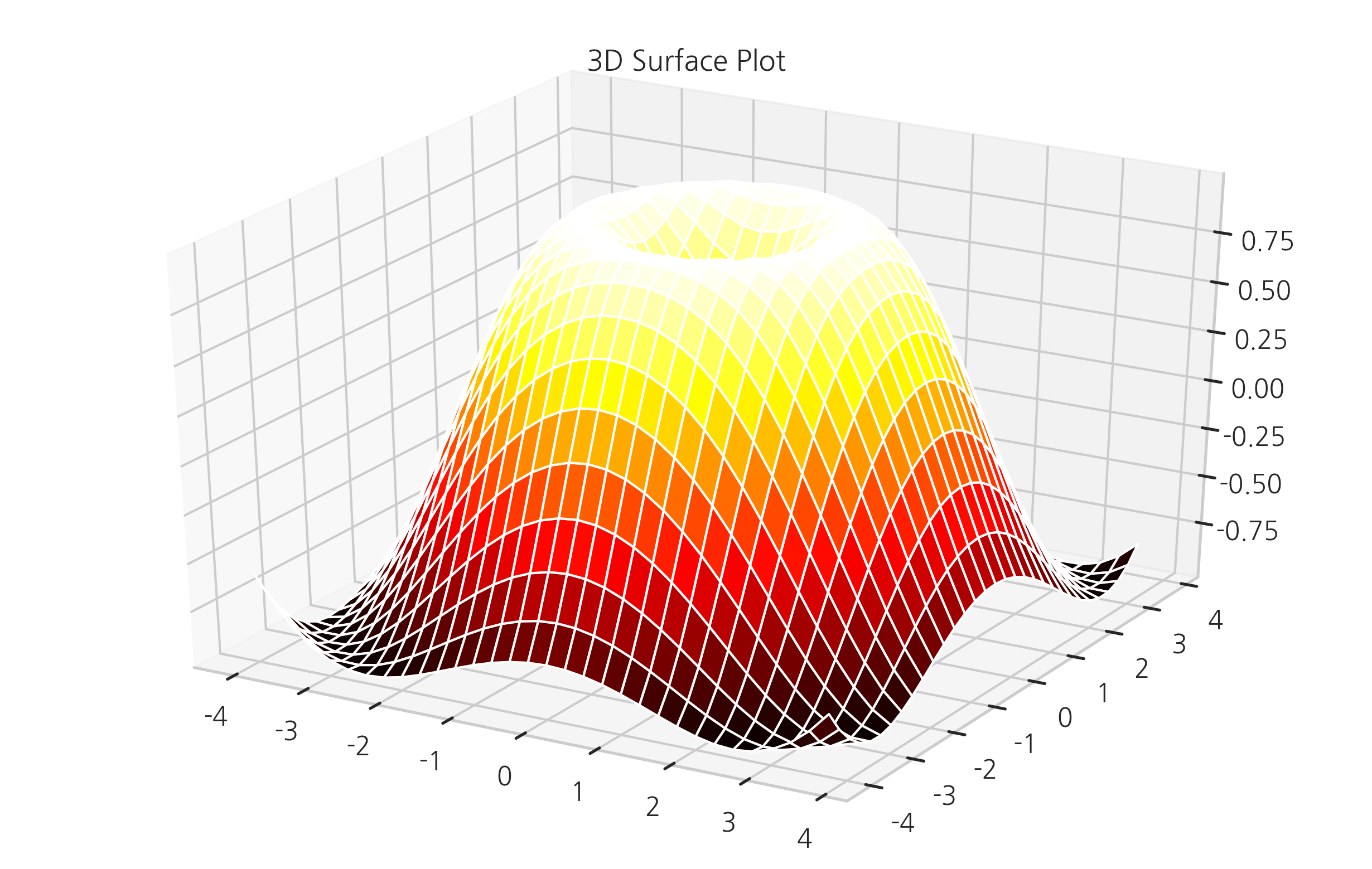