6.4 다중공선성과 변수 선택¶
다중공선성(multicollinearity)란 독립 변수의 일부가 다른 독립 변수의 조합으로 표현될 수 있는 경우이다. 독립 변수들이 서로 독립이 아니라 상호상관관계가 강한 경우에 발생한다. 이는 독립 변수의 공분산 행렬이 full rank 이어야 한다는 조건을 침해한다.
다음 데이터는 미국의 거시경제지표를 나타낸 것이다.
TOTEMP - Total Employment
GNPDEFL - GNP deflator
GNP - GNP
UNEMP - Number of unemployed
ARMED - Size of armed forces
POP - Population
YEAR - Year (1947 - 1962)
스캐터 플롯에서 보듯이 독립변수간의 상관관계가 강하다.
from statsmodels.datasets.longley import load_pandas
dfy = load_pandas().endog
dfX = load_pandas().exog
df = pd.concat([dfy, dfX], axis=1)
sns.pairplot(dfX)
plt.show()
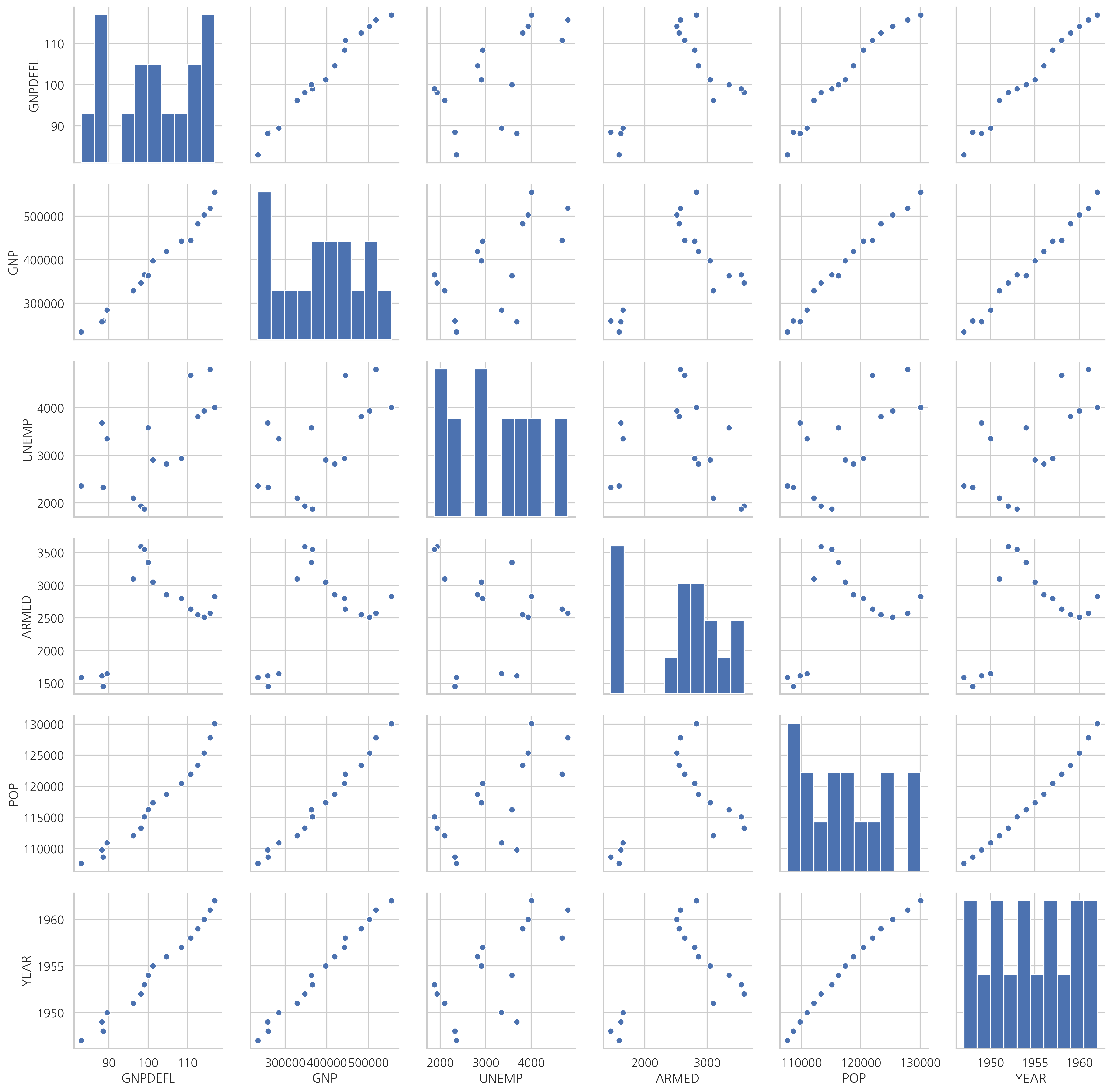
상관관계는 상관계수 행렬로도 살펴볼 수 있다.
dfX.corr()
GNPDEFL | GNP | UNEMP | ARMED | POP | YEAR | |
---|---|---|---|---|---|---|
GNPDEFL | 1.000000 | 0.991589 | 0.620633 | 0.464744 | 0.979163 | 0.991149 |
GNP | 0.991589 | 1.000000 | 0.604261 | 0.446437 | 0.991090 | 0.995273 |
UNEMP | 0.620633 | 0.604261 | 1.000000 | -0.177421 | 0.686552 | 0.668257 |
ARMED | 0.464744 | 0.446437 | -0.177421 | 1.000000 | 0.364416 | 0.417245 |
POP | 0.979163 | 0.991090 | 0.686552 | 0.364416 | 1.000000 | 0.993953 |
YEAR | 0.991149 | 0.995273 | 0.668257 | 0.417245 | 0.993953 | 1.000000 |
cmap = sns.light_palette("darkgray", as_cmap=True)
sns.heatmap(dfX.corr(), annot=True, cmap=cmap)
plt.show()
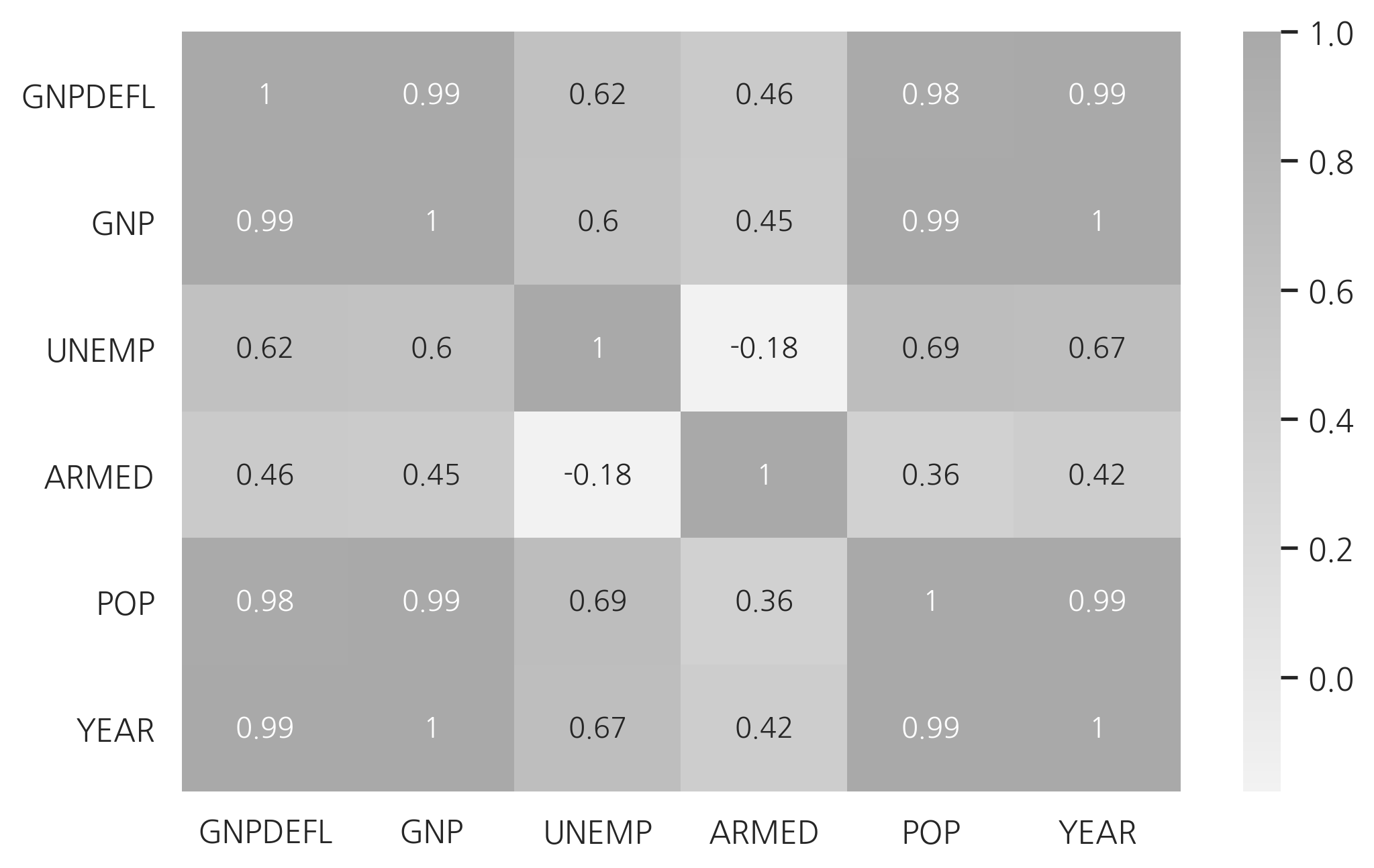
다중 공선성이 있으면 독립변수의 공분산 행렬의 조건수(conditional number)가 증가한다.
from sklearn.model_selection import train_test_split
def get_model1(seed):
df_train, df_test = train_test_split(df, test_size=0.5, random_state=seed)
model = sm.OLS.from_formula("TOTEMP ~ GNPDEFL + POP + GNP + YEAR + ARMED + UNEMP", data=df_train)
return df_train, df_test, model.fit()
df_train, df_test, result1 = get_model1(3)
print(result1.summary())
OLS Regression Results
==============================================================================
Dep. Variable: TOTEMP R-squared: 1.000
Model: OLS Adj. R-squared: 0.997
Method: Least Squares F-statistic: 437.5
Date: Sun, 23 Jun 2019 Prob (F-statistic): 0.0366
Time: 18:13:38 Log-Likelihood: -44.199
No. Observations: 8 AIC: 102.4
Df Residuals: 1 BIC: 103.0
Df Model: 6
Covariance Type: nonrobust
==============================================================================
coef std err t P>|t| [0.025 0.975]
------------------------------------------------------------------------------
Intercept -1.235e+07 2.97e+06 -4.165 0.150 -5e+07 2.53e+07
GNPDEFL 106.2620 75.709 1.404 0.394 -855.708 1068.232
POP 2.2959 0.725 3.167 0.195 -6.915 11.506
GNP -0.3997 0.120 -3.339 0.185 -1.920 1.121
YEAR 6300.6231 1498.900 4.203 0.149 -1.27e+04 2.53e+04
ARMED -0.2450 0.402 -0.609 0.652 -5.354 4.864
UNEMP -6.3311 1.324 -4.782 0.131 -23.153 10.491
==============================================================================
Omnibus: 0.258 Durbin-Watson: 1.713
Prob(Omnibus): 0.879 Jarque-Bera (JB): 0.304
Skew: 0.300 Prob(JB): 0.859
Kurtosis: 2.258 Cond. No. 2.01e+10
==============================================================================
Warnings:
[1] Standard Errors assume that the covariance matrix of the errors is correctly specified.
[2] The condition number is large, 2.01e+10. This might indicate that there are
strong multicollinearity or other numerical problems.
또한 다음처럼 학습용 데이터와 검증용 데이터로 나누어 회귀분석 성능을 비교하면 과최적화가 발생하였음을 알 수 있다.
def calc_r2(df_test, result):
target = df.loc[df_test.index].TOTEMP
predict_test = result.predict(df_test)
RSS = ((predict_test - target)**2).sum()
TSS = ((target - target.mean())**2).sum()
return 1 - RSS / TSS
test1 = []
for i in range(10):
df_train, df_test, result = get_model1(i)
test1.append(calc_r2(df_test, result))
test1
[0.9815050656837723,
0.9738497543069347,
0.9879366369871746,
0.7588861967897188,
0.980720608930437,
0.8937889315168234,
0.8798563810651999,
0.9314665778963799,
0.8608525682180641,
0.9677198735170137]
독립변수가 서로 의존하게 되면 이렇게 과최적화(over-fitting) 문제가 발생하여 회귀 결과의 안정성을 해칠 가능성이 높아진다. 이를 방지하는 방법들은 다음과 같다.
변수 선택법으로 의존적인 변수 삭제
PCA(principal component analysis) 방법으로 의존적인 성분 삭제
정규화(regularized) 방법 사용
VIF¶
다중 공선성을 없애는 가장 기본적인 방법은 다른 독립변수에 의존하는 변수를 없애는 것이다. 가장 의존적인 독립변수를 선택하는 방법으로는 VIF(Variance Inflation Factor)를 사용할 수 있다. VIF는 독립변수를 다른 독립변수로 선형회귀한 성능을 나타낸 것이다. \(i\)번째 변수의 VIF는 다음과 같이 계산한다.
여기에서 \(R^2_i\)는 다른 변수로 \(i\)번째 변수를 선형회귀한 성능(결정 계수)이다. 다른 변수에 의존적일 수록 VIF가 커진다.
StatsModels에서는 variance_inflation_factor
명령으로 VIF를 계산한다.
from statsmodels.stats.outliers_influence import variance_inflation_factor
vif = pd.DataFrame()
vif["VIF Factor"] = [variance_inflation_factor(
dfX.values, i) for i in range(dfX.shape[1])]
vif["features"] = dfX.columns
vif
VIF Factor | features | |
---|---|---|
0 | 12425.514335 | GNPDEFL |
1 | 10290.435437 | GNP |
2 | 136.224354 | UNEMP |
3 | 39.983386 | ARMED |
4 | 101193.161993 | POP |
5 | 84709.950443 | YEAR |
상관계수와 VIF를 사용하여 독립 변수를 선택하면 GNP, ARMED, UNEMP 세가지 변수만으로도 비슷한 수준의 성능이 나온다는 것을 알 수 있다.
def get_model2(seed):
df_train, df_test = train_test_split(df, test_size=0.5, random_state=seed)
model = sm.OLS.from_formula("TOTEMP ~ scale(GNP) + scale(ARMED) + scale(UNEMP)", data=df_train)
return df_train, df_test, model.fit()
df_train, df_test, result2 = get_model2(3)
print(result2.summary())
OLS Regression Results
==============================================================================
Dep. Variable: TOTEMP R-squared: 0.989
Model: OLS Adj. R-squared: 0.981
Method: Least Squares F-statistic: 118.6
Date: Sun, 23 Jun 2019 Prob (F-statistic): 0.000231
Time: 18:13:39 Log-Likelihood: -57.695
No. Observations: 8 AIC: 123.4
Df Residuals: 4 BIC: 123.7
Df Model: 3
Covariance Type: nonrobust
================================================================================
coef std err t P>|t| [0.025 0.975]
--------------------------------------------------------------------------------
Intercept 6.538e+04 163.988 398.686 0.000 6.49e+04 6.58e+04
scale(GNP) 4338.7051 406.683 10.669 0.000 3209.571 5467.839
scale(ARMED) -812.1407 315.538 -2.574 0.062 -1688.215 63.933
scale(UNEMP) -1373.0426 349.316 -3.931 0.017 -2342.898 -403.187
==============================================================================
Omnibus: 0.628 Durbin-Watson: 2.032
Prob(Omnibus): 0.731 Jarque-Bera (JB): 0.565
Skew: 0.390 Prob(JB): 0.754
Kurtosis: 1.958 Cond. No. 4.77
==============================================================================
Warnings:
[1] Standard Errors assume that the covariance matrix of the errors is correctly specified.
다중공선성을 제거한 경우에는 학습 성능과 검증 성능간의 차이가 줄어들었음을 확인할 수 있다. 즉, 과최적화가 발생하지 않는다.
test2 = []
for i in range(10):
df_train, df_test, result = get_model2(i)
test2.append(calc_r2(df_test, result))
test2
[0.9763608388904907,
0.9841984331185702,
0.9687069366140136,
0.9397304053201785,
0.9773357061188462,
0.9561262155732316,
0.980385249669863,
0.9917361722470804,
0.9837134067639467,
0.9789512977093212]
plt.subplot(121)
plt.plot(test1, 'ro', label="검증 성능")
plt.hlines(result1.rsquared, 0, 9, label="학습 성능")
plt.legend()
plt.xlabel("시드값")
plt.ylabel("성능(결정계수)")
plt.title("다중공선성 제거 전")
plt.ylim(0.5, 1.2)
plt.subplot(122)
plt.plot(test2, 'ro', label="검증 성능")
plt.hlines(result2.rsquared, 0, 9, label="학습 성능")
plt.legend()
plt.xlabel("시드값")
plt.ylabel("성능(결정계수)")
plt.title("다중공선성 제거 후")
plt.ylim(0.5, 1.2)
plt.suptitle("다중공선성 제거 전과 제거 후의 성능 비교", y=1.04)
plt.tight_layout()
plt.show()
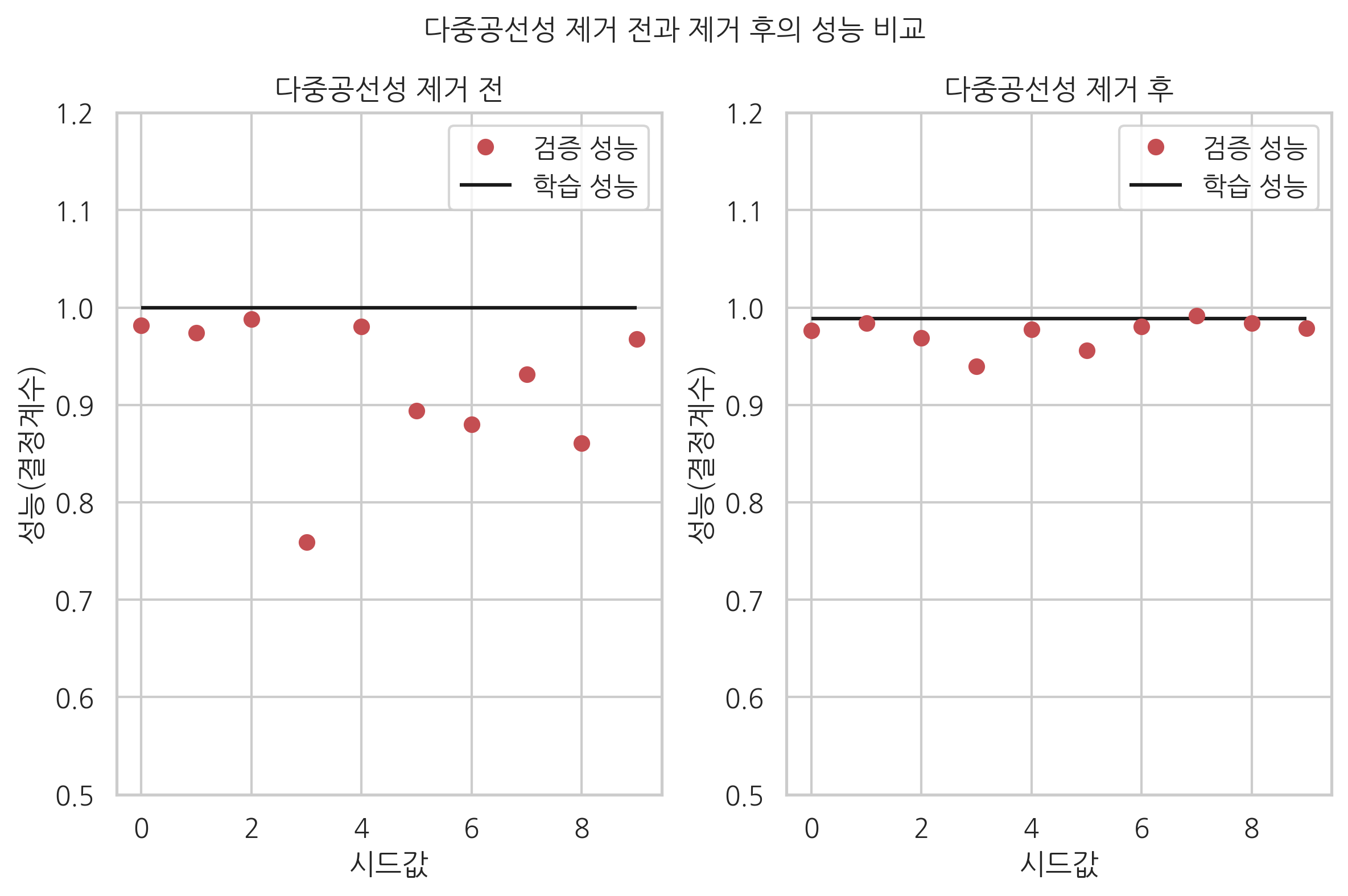
보스턴 집값 예측 문제에 응용¶
from sklearn.datasets import load_boston
boston = load_boston()
dfX0 = pd.DataFrame(boston.data, columns=boston.feature_names)
from patsy import dmatrix
formula = "scale(CRIM) + scale(I(CRIM ** 2)) + " + \
"scale(ZN) + scale(I(ZN ** 2)) + scale(INDUS) + " + \
"scale(NOX) + scale(RM) + scale(AGE) + " + \
"scale(np.log(DIS)) + scale(RAD) + scale(TAX) + " + \
"scale(np.log(PTRATIO)) + scale(B) + scale(np.log(LSTAT)) + CHAS"
dfX = dmatrix(formula, dfX0, return_type="dataframe")
dfy = pd.DataFrame(boston.target, columns=["MEDV"])
idx_outlier = \
np.array([7, 54, 148, 152, 160, 214, 253, 267, 364, 365, 367, 368, 369,
371, 372, 374, 380, 385, 397, 398, 399, 400, 401, 405, 409, 410,
412, 413, 414, 415, 416, 418, 419, 426, 445, 489, 490, 492, 505,
161, 162, 163, 166, 186, 195, 204, 225, 257, 267, 283, 368, 369,
370, 371, 372])
idx = list(set(range(len(dfX))).difference(idx_outlier))
dfX = dfX.iloc[idx, :].reset_index(drop=True)
dfy = dfy.iloc[idx, :].reset_index(drop=True)
cmap = sns.light_palette("black", as_cmap=True)
sns.heatmap(dfX.corr(), annot=True, fmt='3.1f', cmap=cmap)
plt.show()
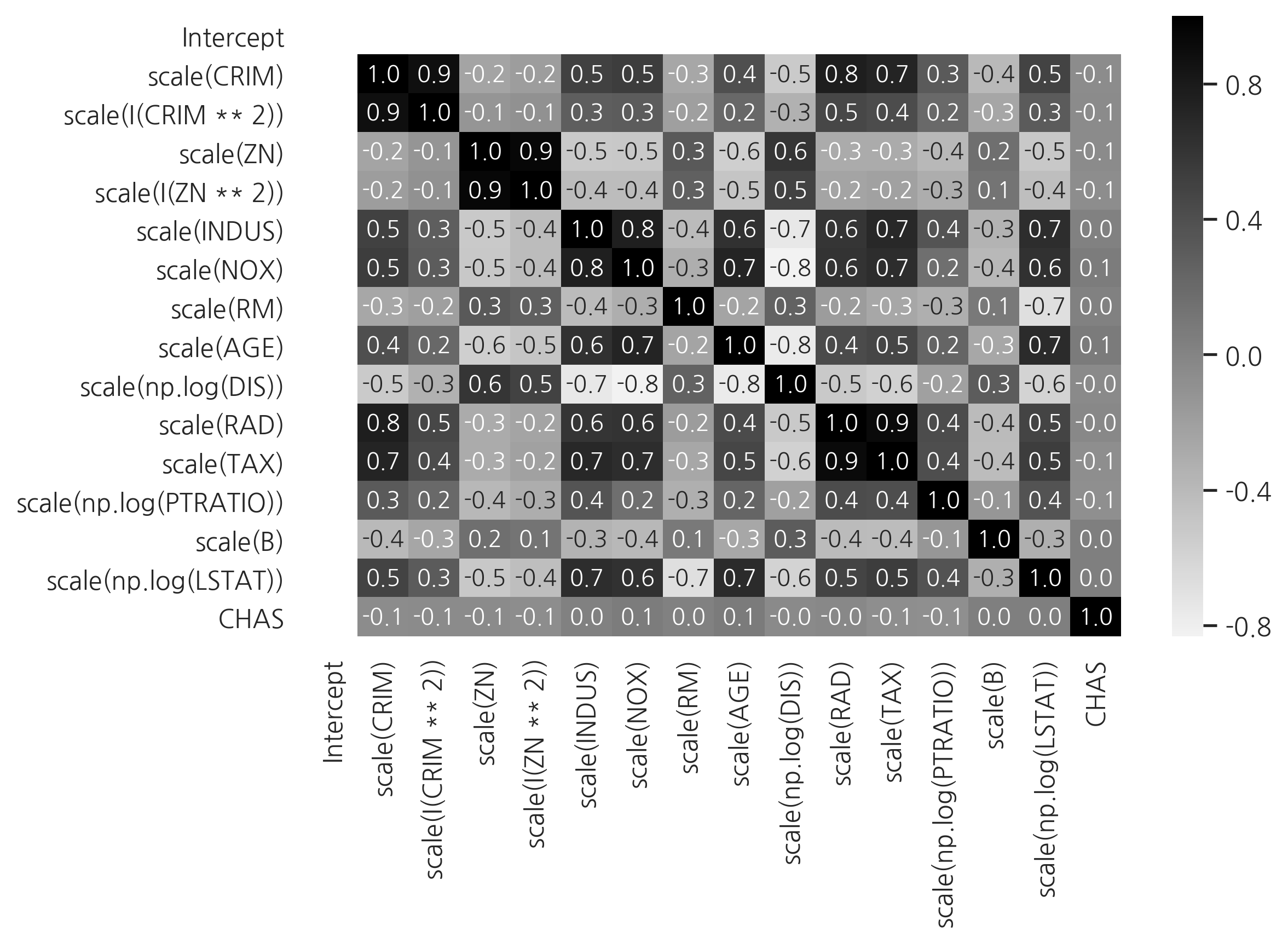
vif = pd.DataFrame()
vif["VIF Factor"] = [variance_inflation_factor(
dfX.values, i) for i in range(dfX.shape[1])]
vif["features"] = dfX.columns
vif = vif.sort_values("VIF Factor").reset_index(drop=True)
vif
VIF Factor | features | |
---|---|---|
0 | 1.061624 | CHAS |
1 | 1.338325 | scale(B) |
2 | 1.478553 | Intercept |
3 | 1.780320 | scale(np.log(PTRATIO)) |
4 | 2.596496 | scale(RM) |
5 | 3.748931 | scale(AGE) |
6 | 3.807459 | scale(INDUS) |
7 | 4.682812 | scale(np.log(LSTAT)) |
8 | 5.071802 | scale(NOX) |
9 | 5.215025 | scale(np.log(DIS)) |
10 | 9.107858 | scale(TAX) |
11 | 10.218588 | scale(I(CRIM ** 2)) |
12 | 11.254736 | scale(RAD) |
13 | 11.751869 | scale(I(ZN ** 2)) |
14 | 14.646056 | scale(ZN) |
15 | 21.260182 | scale(CRIM) |
model_boston1 = sm.OLS(np.log(dfy), dfX)
result_boston1 = model_boston1.fit()
print(result_boston1.summary())
OLS Regression Results
==============================================================================
Dep. Variable: MEDV R-squared: 0.872
Model: OLS Adj. R-squared: 0.868
Method: Least Squares F-statistic: 199.9
Date: Sun, 23 Jun 2019 Prob (F-statistic): 1.56e-185
Time: 18:13:41 Log-Likelihood: 317.45
No. Observations: 456 AIC: -602.9
Df Residuals: 440 BIC: -536.9
Df Model: 15
Covariance Type: nonrobust
==========================================================================================
coef std err t P>|t| [0.025 0.975]
------------------------------------------------------------------------------------------
Intercept 3.0338 0.007 433.880 0.000 3.020 3.048
scale(CRIM) -0.3471 0.044 -7.976 0.000 -0.433 -0.262
scale(I(CRIM ** 2)) 0.3075 0.071 4.331 0.000 0.168 0.447
scale(ZN) -0.0465 0.022 -2.110 0.035 -0.090 -0.003
scale(I(ZN ** 2)) 0.0440 0.020 2.206 0.028 0.005 0.083
scale(INDUS) 0.0037 0.012 0.323 0.747 -0.019 0.026
scale(NOX) -0.0652 0.013 -5.001 0.000 -0.091 -0.040
scale(RM) 0.0999 0.011 9.195 0.000 0.079 0.121
scale(AGE) -0.0273 0.011 -2.438 0.015 -0.049 -0.005
scale(np.log(DIS)) -0.1008 0.014 -7.368 0.000 -0.128 -0.074
scale(RAD) 0.1634 0.020 8.106 0.000 0.124 0.203
scale(TAX) -0.0934 0.018 -5.153 0.000 -0.129 -0.058
scale(np.log(PTRATIO)) -0.0699 0.008 -8.872 0.000 -0.085 -0.054
scale(B) 0.0492 0.007 6.699 0.000 0.035 0.064
scale(np.log(LSTAT)) -0.1487 0.013 -11.074 0.000 -0.175 -0.122
CHAS 0.0659 0.026 2.580 0.010 0.016 0.116
==============================================================================
Omnibus: 28.653 Durbin-Watson: 1.309
Prob(Omnibus): 0.000 Jarque-Bera (JB): 43.266
Skew: 0.465 Prob(JB): 4.03e-10
Kurtosis: 4.188 Cond. No. 35.2
==============================================================================
Warnings:
[1] Standard Errors assume that the covariance matrix of the errors is correctly specified.
cols = ["Intercept", "CHAS", "scale(B)", "scale(CRIM)",
"scale(np.log(PTRATIO))", "scale(RM)", "scale(np.log(LSTAT))"]
model_boston2 = sm.OLS(np.log(dfy), dfX[cols])
result_boston2 = model_boston2.fit()
print(result_boston2.summary())
OLS Regression Results
==============================================================================
Dep. Variable: MEDV R-squared: 0.836
Model: OLS Adj. R-squared: 0.834
Method: Least Squares F-statistic: 380.7
Date: Sun, 23 Jun 2019 Prob (F-statistic): 1.42e-172
Time: 18:13:41 Log-Likelihood: 260.52
No. Observations: 456 AIC: -507.0
Df Residuals: 449 BIC: -478.2
Df Model: 6
Covariance Type: nonrobust
==========================================================================================
coef std err t P>|t| [0.025 0.975]
------------------------------------------------------------------------------------------
Intercept 3.0192 0.007 445.252 0.000 3.006 3.033
CHAS 0.0884 0.028 3.141 0.002 0.033 0.144
scale(B) 0.0558 0.008 6.989 0.000 0.040 0.072
scale(CRIM) -0.1179 0.013 -9.120 0.000 -0.143 -0.092
scale(np.log(PTRATIO)) -0.0508 0.007 -6.936 0.000 -0.065 -0.036
scale(RM) 0.1153 0.011 10.828 0.000 0.094 0.136
scale(np.log(LSTAT)) -0.1570 0.011 -14.179 0.000 -0.179 -0.135
==============================================================================
Omnibus: 29.141 Durbin-Watson: 1.113
Prob(Omnibus): 0.000 Jarque-Bera (JB): 42.637
Skew: 0.483 Prob(JB): 5.51e-10
Kurtosis: 4.145 Cond. No. 5.91
==============================================================================
Warnings:
[1] Standard Errors assume that the covariance matrix of the errors is correctly specified.